Revolutionizing Agriculture: Utilizing Machine Learning Datasets for Enhanced Outcomes
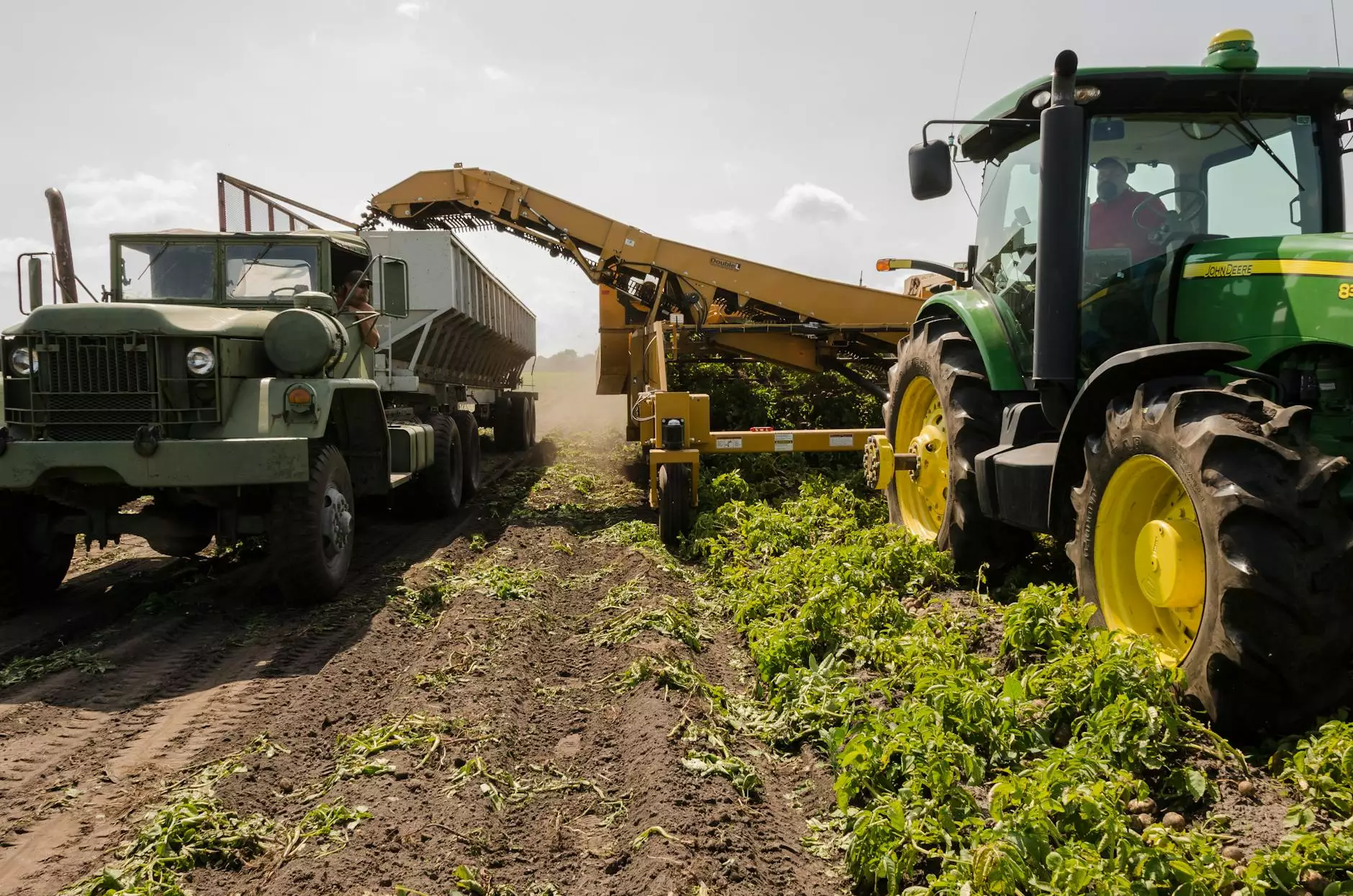
In today’s fast-paced world, technology continues to transform various industries, and one of the most significant impacts is seen in agriculture. The integration of machine learning into agricultural practices holds the promise of optimizing outputs, enhancing productivity, and ensuring sustainability. Central to this transformation is the availability and effective utilization of agriculture datasets for machine learning.
Understanding the Role of Machine Learning in Agriculture
The agricultural sector is under constant pressure to meet the demands of a growing population while ensuring environmental protection. Machine Learning (ML) serves as a pivotal tool in achieving these goals. By analyzing large volumes of data, ML algorithms can uncover patterns and insights that were previously unimaginable. This leads to data-driven decision-making that significantly enhances productivity.
What are Agriculture Datasets?
Agriculture datasets consist of structured and unstructured data collected from various agricultural activities. This data can include:
- Soil health parameters
- Crop yield data
- Weather conditions
- Pest and disease prevalence
- Water usage statistics
- Market prices for crops
The vast range of datasets allows farmers and agricultural scientists to comprehensively analyze the factors influencing crop production and resource management.
Types of Agriculture Datasets
There are several types of datasets that are crucial for machine learning applications in agriculture, including:
- Remote Sensing Data: Captured using satellites and drones, this data helps in monitoring crop health, soil moisture, and land use changes.
- Sensor Data: IoT devices and sensors provide real-time data on environmental parameters, critical for precise farming.
- Market Data: This consists of historical and real-time data on crop prices, which helps farmers make better sales decisions.
- Genetic Data: Information on crop genetics can be used to predict the best African varieties for specific conditions.
Why Are Agriculture Datasets Essential for Machine Learning?
The effectiveness of machine learning models largely depends on the quality and volume of the datasets used. In the context of agriculture, high-quality datasets enable farmers to tackle challenges such as disease outbreaks, pest invasions, and climate variability. Below are several reasons why these datasets are essential:
1. Data-Driven Decision Making
The availability of comprehensive datasets aids farmers in making informed decisions. For instance, utilizing agriculture datasets for machine learning can help predict the optimal time for planting or harvesting. By analyzing weather patterns and soil conditions, farmers can improve their productivity and reduce waste.
2. Precision Agriculture
Precision agriculture relies heavily on data analysis to optimize field-level management. Data collected from various sensors can inform targeted interventions, ensuring that resources such as water and fertilizers are used efficiently. This approach not only enhances productivity but also minimizes environmental impact.
3. Predictive Analytics
Machine learning models can analyze past data to predict future outcomes. For example, by examining historical yield data alongside weather patterns, farmers can predict the performance of their crops. These insights facilitate better planning and resource allocation.
4. Improved Plant Breeding
Machine learning algorithms are increasingly used in plant breeding programs. By analyzing genetic datasets, researchers can identify traits associated with higher yields and disease resistance. This accelerates the development of new, improved crop varieties.
Challenges in Utilizing Agriculture Datasets for Machine Learning
Despite the advantages, the integration of machine learning in agriculture faces several challenges:
1. Data Quality and Availability
Not all datasets are created equal. Incomplete, outdated, or inaccurate data can lead to flawed models and outputs. Reliable data sources and collection methods must be established to ensure high-quality datasets.
2. Technology Adoption Barriers
Smallholder farmers may lack access to modern technology and training required for implementing machine learning solutions. This technological gap can hinder the widespread adoption of beneficial practices.
3. Ethical Considerations
The use of data raises ethical concerns, particularly regarding privacy and data ownership. It is essential for stakeholders in agriculture to establish clear policies that protect individuals and promote equitable data sharing.
Applications of Machine Learning in Agriculture
As the agricultural sector embraces technology, several applications of machine learning are emerging:
1. Crop Management
Machine learning algorithms are being used to monitor crops consistently. They analyze data from various sources to provide recommendations on irrigation schedules, pest control measures, and fertilization regimes.
2. Yield Prediction
By incorporating various datasets, machine learning models can accurately forecast crop yields, helping farmers plan their resources and distribution strategies effectively.
3. Soil Health Monitoring
Understanding soil health is crucial for sustainable agriculture. Machine learning can process soil data to detect nutrient deficiencies and recommend corrective actions.
4. Pest Detection
Using machine vision and deep learning, it is now possible to identify pests in crops early on, enabling timely interventions and reducing the reliance on pesticides.
Conclusion
The integration of agriculture datasets for machine learning represents a paradigm shift in modern agriculture. By leveraging technology, farmers can optimize operations, enhance productivity, and address sustainability challenges more effectively than ever before. However, to harness the full potential of these datasets, challenges such as data quality, technology adoption, and ethical considerations must be carefully managed. As we move forward, the collaboration between technology providers, researchers, and the farming community will be essential to realizing a more productive and sustainable agricultural future.
Get Started with Agriculture Datasets
If you are ready to explore the world of agriculture datasets for machine learning, several resources are available to help you get started:
- Research institutions often provide access to agricultural datasets.
- Online platforms and data repositories can offer a variety of datasets for experimentation.
- Engagement with community forums and agricultural technology providers can provide insights and support.
As you embark on your journey into the intersection of agriculture and technology, remember that the future of farming lies in data-driven innovation and sustainability.
agriculture dataset for machine learning